What role do misfolded proteins play in neurological diseases?
Introduction to the Dynamics of Misfolded Proteins
Proteins are large molecules composed of amino acids and, their correct folding configuration provides long-term stability to biological environments. In contrast, a failure of proper folding is commonly associated with a variety of pathological conditions (Dobson, 2003; Nassar, 2021). Hence, proteins that fail to configure properly are called misfolded proteins (MP) and are thought to be the initial process that triggers multiple disorders, such as Alzheimer’s disease (AD), Parkinson’s disease (PD), Amyotrophic Lateral Sclerosis (ALS), Creutzfeldt-Jakob disease (CJD), and several other human neurodegenerative disorders (Scheckel, 2018; Mehra, 2019; Lövestam, 2023; Michaels, 2023).
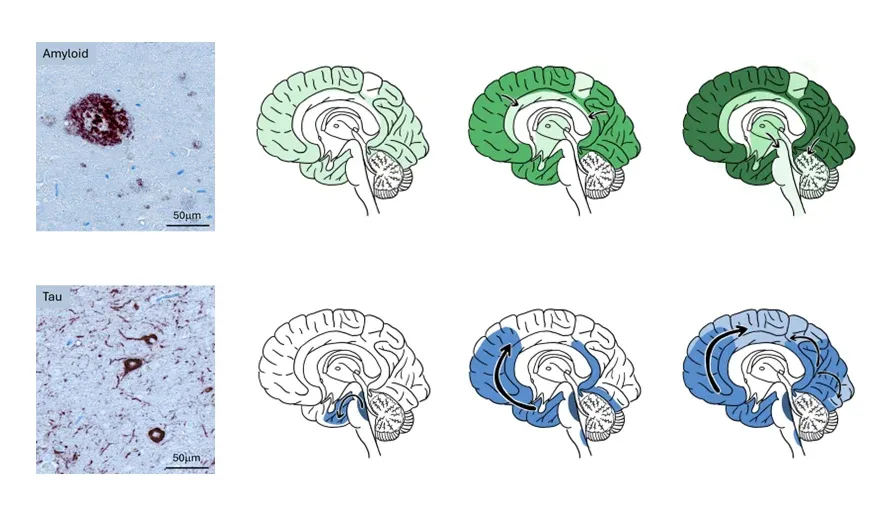
Alzheimer’s Disease is characterized by different microscopic patterns of Aß and tau misfolded proteins that yields characteristic anatomical progression patterns of Aß and tau aggregates in the cortex.
There is a series of intrinsic mechanisms surrounding the misfolding protein process that need to be fully characterized in order to gain a full understanding of their toxicity effect in cells (Eisele, 2016; Lewis, 2016). Such mechanisms include MP seeding, aggregation, propagation and/or spreading. Thus, the specific signatures in the time course of these MP-related mechanisms are usually referred as the MP dynamics. The main obstacle towards the identification of conformational disease-specific triggering events is the ability to extrapolate the impact of the MP dynamics at the molecular level to more macroscopic scales.
Mathematical Models for Misfolded Proteins
Mathematical models are theoretical environments that aim to simulate and reproduce as much as possible the true dynamics of biological processes. Mathematical models expressed by Differential Equations are a natural choice since they can easily reproduce nonlinear and complex dynamics of several time-interacting processes. In the context of misfolding proteins, mathematical models can be categorized into two broad classes. A class of models aimed to simulate molecular-level processes like seeding, aggregation and short-range cell-to-cell spatial spreading, and a class of models that simulate more macroscopic interactions like long-range MP propagation within the human brain. Provided such a categorization, one can find that the microscopic scale type of models has been mainly developed within the field of chemical kinetics. In contrast, the larger scale (i.e. macroscopic) type of models has mostly proposed within the framework of neuroimaging studies.
Prion-like Dynamics
Since prion disease can be also considered a MP process (Scheckel, 2018), it was natural that the pioneering mathematical models for MP appeared to simulate the biological mechanisms of prion replication and aggregation (Eigen, 1996). Based on Ordinary Differential Equations (ODEs), the initial prototype model that was broadly accepted is currently known as the Nucleated Polymerization Model (NPM) (Nowak, 1998) and focused on autocatalysis mechanisms that intended to explain the conversion of a typical prion protein into an infectious agent protein. Remarkably, Nowak et al. (Nowak, 1998) realized that the mechanisms of protein replication and aggregation included in the NPM were analogous to the dynamics found in popular epidemiological models for describing virus dynamics. Thus, it is not a surprise that the modern macroscopic models to describe MP propagation have also been inspired in well-founded epidemiological models.
By establishing the NPM for prion dynamics as a foundation stone, several studies (Petkova, 2005; Frost, 2009) have demonstrated that fibril aggregates of α-synuclein, tau, and Aß proteins self-propagate under biochemical mechanisms analogous to those described for prion aggregation/propagation. These observations, in conjunction with several in vivo animal models, established the founding of the so-called prion-like hypothesis of neurodegenerative disease progression (Frost, 2010). Henceforth, under the prion-like hypothesis, the MP “infectivity” propagates from initial seed regions with a relative high concentration of pathogenic proteins to other “non-infected” brain regions.
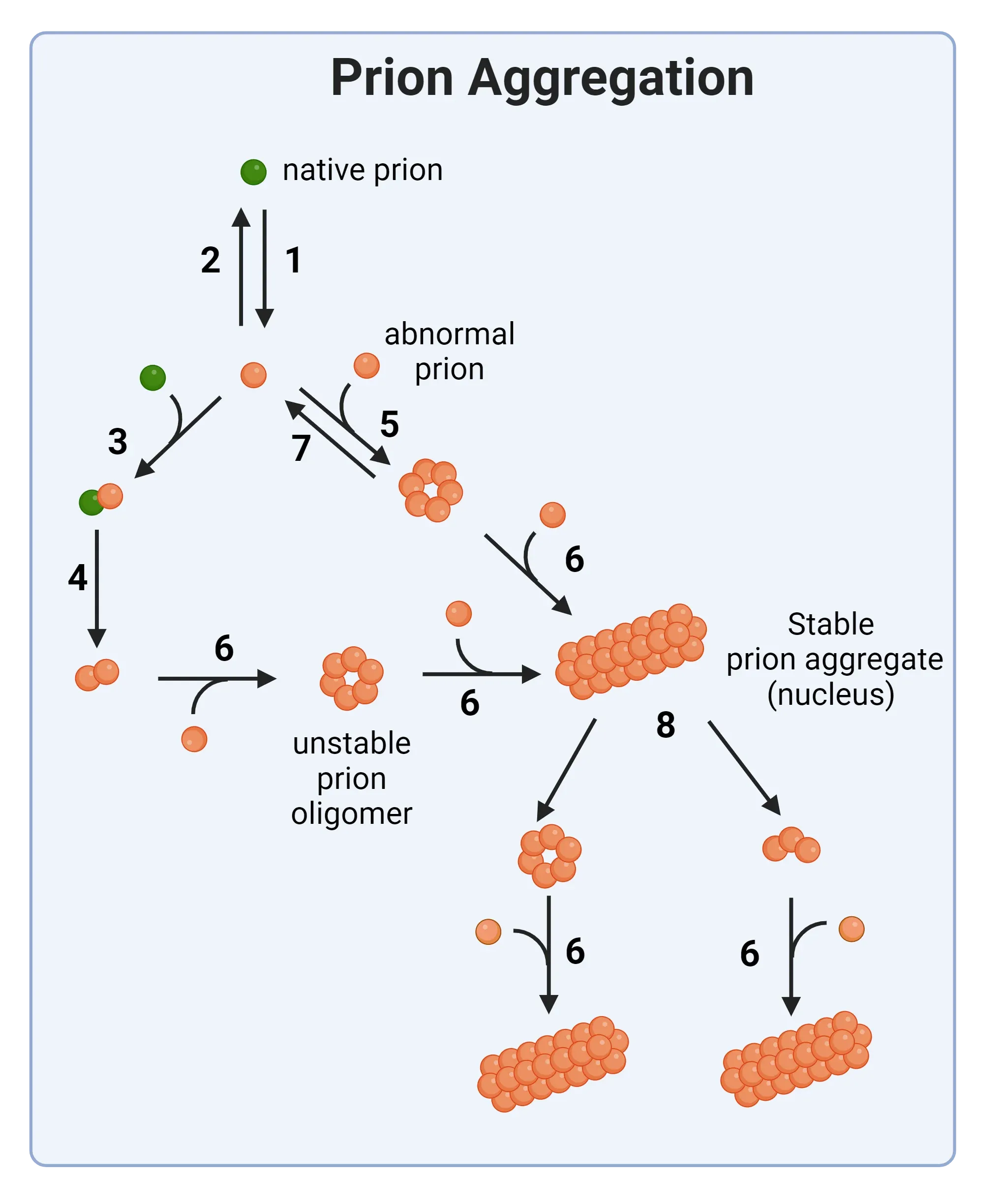
Schematic representation of prion aggregation mechanisms. Native prion molecules undergo conformational changes that lead to an abnormal configuration (Step 1). From there, several seeding and aggregation steps show the formation of unstable prion oligomers to finally produce stable prior aggregates.
MP Propagation: Spatial Spreading
A breakthrough in the study of the MP dynamics was given by Payne et al. (Payne, 1998) who provided a plausible explanation to the latency between the appearance of infectious agent protein in the Peripherical Nervous System (PNS) and its appearance in the Central Nervous System (CNS), which had been previously observed in animal models (Scott, 1992). Thus, Payne et al. (Payne, 1998) proposed the hypothesis that such observed latency was more likely related to the rate of spread between neighboring localities, rather than explained by the rate at which a typical prion protein is converted into infectious agent protein at a specific spatial location. To prove such a hypothesis, the original epidemiological-like configuration of the NPM needed to be extended to accommodate spatial “connectivity” features into the temporal evolution of prion kinetics (Stumpf, 2000). The main underlying assumption in this type of extended model was that the infectious agent protein spatially spreads along axons and dendrites by slow axonal transport. Additionally, it was natural to also assume that the rate of spread from cell to cell depends on the connectivity strengths.
How are the dynamics of misfolded proteins mathematically modeled?
Chemical Kinetics to Model Protein Aggregation Mechanisms
The most modern approach to model MP is to use principles of chemical kinetics to derive master equations that explicitly account for the different microscopic processes involved in the protein’s aggregation mechanisms (Cohen, 2012; Cohen, 2013). The goal is to characterize the kinetics of protein aggregation with integrated rate laws that could be derived from such master equations.
By considering filamentous growth phenomena and under the basic principles of homogenous nucleation, growth, and dissociation processes, Oosawa et al. (Oosawa, 1962) proposed the first prototype of master equations models for protein aggregation. As in the case of the NPM, the master equations can be analytically described by an infinite set of ODEs. However, in practice, such an infinite set of ODEs yields to a closed system of only two ODEs that are commonly called the moment equations and characterize the temporal evolution of the number and mass concentrations of the protein aggregates, which are quantities that relate directly to measurements of most common in vitro experiments. The desired integrated rate laws can then straightforwardly be obtained by solving the moment equations (Cohen, 2013). However, analytically solving the moment equations is a complicated task, particularly for the entire reaction time course.
A major contribution to the master equations theory for protein formation was then provided by Knowles et al. (Knowles, 2009), who generated analytical solutions for the integrated rate law that were valid for the entire reaction time course. As in the case of the original Oosawa’s theory, these explicit integrated rate laws can characterize the dependency of the reaction time course on two parameters that can be easily related to experimentally observed phenomenological variables (Cohen, 2011). From a practical point of view, it is extremely important to have an analytical formula for the integrated rate laws, which would allow to fit models with data coming from different experimental conditions. For instance, the model proposed by Knowles et al. (Knowles, 2009) was fitted to in vitro data corresponding to the peptides Aß40 and Aß42, providing a clearer understanding about the formation of Aß aggregates (Meisl, 2014; Meisl, 2016). During the last few years, the master equations approach and the corresponding integrated rate laws have been the subject of multiple extensions and generalizations. A remarkable one was provided by Cohen et al. (Cohen, 2014), which, by including mechanisms of spatial propagation in the master equations, found that a secondary aggregation pathway governs the speed of spatial propagation by diffusion.
Coagulation Theory for Aggregation
As in the case of the master equations approach, the coagulation theory described by Smoluchowski’s equations also covers the general case of self-association among particles assemblies of different sizes. The first references to Smoluchowski’s equations in the context of protein aggregation appeared in Murphy et al. (Murphy, 2000) for describing the axial elongation of fibrils by end-to-end aggregation of shorter fibrils. Likewise, by using Smoluchowski’s equations, Craft et al. (Craft, 2005) proposed a polymerization model where the nucleation process appears implicitly incorporated within the mechanism of association of small size polymers (e.g. monomers, micelles, filaments).
An important turning point in the field of protein aggregation and propagation mechanisms modeling is due to Achdou et al. (Achdou, 2013). Rather than working with moment equations, Achdou et al. (Achdou, 2013) truncated the infinite set of ODEs at a large enough number N. In this way, the equation corresponding to the truncation number N should be able to describe the time evolution of the summary of all protein assemblies composed of more than N monomers. Additionally, Achdou et al. (Achdou, 2013) realized that the Smoluchowski’s equations also provide a straightforward framework for incorporating processes of spatial propagation.
The development of modern imaging techniques demands the possibility to probe models at scales greater than microscopic scales. With this aim, a large-scale model was proposed by Bertsch et al. (Bertsch, 2017), which coupled a set of truncated Smoluchowski’s equations to a kinetic-type transport equation that models the spreading of neuronal damage by neuron-to-neuron prion-like transmission. Such a modeling then has the ability to incorporate two different temporal scales evolving over the same spatial domain: a rapid temporal scale (e.g. minutes, hours) for microscopic processes (by Smoluchowski’s equations); and a slow (e.g. months, years) scale to account for the observed progression of AD in humans (by the transport equation).
Modeling Interregional Propagation with the Network Approach
Modeling spatial spreading of misfolded proteins by a homogeneous diffusion process is not a realistic choice in large spatial domains, such as the whole brain. In contrast to a homogenous diffusional spread, the network approach models a rapid infection spread within clusters of highly connected neurons, and propagation to other clusters via long-distance connections (Matthäus, 2006). Hence, by using a discrete Susceptible-Infected (SI) epidemiological model, Matthäus et al. (Matthäus, 2006) characterized the spread of prion protein infection along a network of inter-connected neurons. More importantly, this network-based mathematical framework can be easily adapted to describe a greater scale scenario where the network nodes represent distant regions covering large spatial domains.
In this line, Raj et al. (Raj, 2012) proposed a macroscopic Network Diffusion Model (NDM), where the number of MP afferents from a given brain region to any other region uniquely depends upon the disease concentration factors in both regions and upon the anatomical connection strength between them. By considering a more realistic scenario, Iturria-Medina et al. (Iturria-Medina, 2014) introduced an Epidemic Spreading Model (ESM) that simultaneously accounts for the regional capacity to produce/clean MPs and the topological information of the brain’s anatomical network. When applied to the study of late onset AD using Amyloid PET data, this model was able to reproduce Aß deposition patterns at the individual level. The existence of detailed pathological mechanisms and hypotheses for AD progression has motivated the consideration of a more integrative multi-factorial modeling approach that extends beyond the misfolded protein formation and propagation (Edelstein-Keshet, 2002; Luca, 2003).
How can theoretical models for therapeutic interventions be used?
Typically, mathematical models of proteins aggregation are used as a surrogate to therapeutics interventions in vitro. The general idea is to simulate how a therapeutic intervention (e.g. drugs, antibodies, molecular chaperones) may inhibit certain microscopic aggregation processes (Arosio, 2014; Nasica-Labouze, 2015; Kulenkampff, 2021; Ghosh, 2023). Then kinetic rates of protein aggregation can be estimated and compared under both natural and inhibition conditions. It proceeds by firstly assuming a hypothetical change (e.g. by treatment) on the appropriate kinetic rates and then substituting those modified rates into the original model to simulate post-treatment states.
Monitoring kinetic rates as function of a hypothetical drug dose might help to extrapolate small drug dosages inherent to in vitro environments to dosages more closely resembling in vivo conditions (Masel, 2000). In their pioneering study, Masel et al. (Masel, 2000) used mathematical models to simulate the inhibition of amyloid propagation with three main approaches: (i) by lowering the effective monomer concentration of unfolded proteins; (ii) by blocking growing polymer ends; and (iii) by increasing the polymer breakage rate. They found that therapeutics following the second strategy would provide promising results, while the remaining ones may be ineffective or even accelerate the amyloid formation process at low drug dosages.
Simulated inhibition of amyloid aggregation/propagation by three different strategies: (1) lowering the effective monomer concentration, (2) blocking growing polymer ends, and (3) increasing the polymer breakage rate.
As another example, in the context of amyloid-beta protein, Craft et al. (Craft, 2005) established a nonlinear relationship between the polymerization ratio and the total Aß burden. Since the polymerization ratio depends on four different kinetic rates, several treatment strategies could be tested in this context: (i) the reduction of Aß monomers production rate, (ii) the enhancement of fragmentation, (iii) the enhancement of the clearance (i.e. degradation) rate, and (iv) the reduction of the elongation rate. By simulation of the above-mentioned scenarios, Craft et al. (Craft, 2005) concluded that any drug treatment based on clearance rate enhancers might be more effective in reducing the total Aß burden than those based on polymer fragmentation enhancers.
More recently, Arosio et al. (Arosio, 2016) used the chemical kinetic approach to model the interaction between molecular chaperones and different protein species. The main idea was to identify which microscopic reaction steps (i.e. primary nucleation, elongation, fragmentation, and secondary nucleation) were perturbed by the binding of the molecular chaperones to certain protein species. By using the master equations approach, Arosio et al. (Arosio, 2016) compared the estimated kinetic rate constants in the absence and presence of different concentrations of molecular chaperones. For the case of the Aß42 protein, such analysis revealed that the action of molecular chaperone termed DNAJB6 inhibits the primary nucleation process. In contrast, the presence of another molecular chaperone (termed proSP-C Brichos) produces a reduction in the secondary nucleation rate.
As a further example, Habchi et al. (Habchi, 2016) used the chemical kinetics approach for developing a rational drug discovery strategy and reported that an anticancer drug (termed bexarotene) disturbs the primary nucleation step in the Aß42 aggregation, delays the formation of toxic oligomers, and completely suppresses Aß42 deposition. This general framework yields a systematic drug discovery strategy aimed to identify a variety of small molecules (Habchi, 2017) and antibodies (Aprile, 2017) that not only target the onset of aggregation, but also the secondary nucleation step responsible for the proliferation of toxic Aß42 oligomers.
Recent advances in the field of chemical kinetics and protein aggregation have undoubtedly yielded a renewed interest in therapeutic intervention modeling as a valuable tool for the discovery of new potential therapeutic agents in MP-related diseases (Linse, 2020; Kulenkampff, 2021; Ghosh, 2023).
Our team would be happy to answer any questions about Modeling Protein Misfolding in Neurological Diseases or provide specific information about our other Imaging services.
Discover more about our Imaging Services
Related Content
Up-to-date information on best practices related to the use of neuroimaging in clinical trials of neurological diseases.
PET Imaging in Huntington’s Disease Clinical Trials
An overview of the use of PET imaging biomarkers for Huntington’s disease (HD) clinical trials.
Longitudinal Change in Tau PET in MCI & Alzheimer’s Disease
An overview of the natural history of change in Tau PET tracer uptake & binding in Mild Cognitive Impairment (MCI) & Alzheimer’s disease (AD).
Imaging Biomarkers to Distinguish CBD from Other Tauopathies
Overview of brain imaging biomarkers to identify Corticobasal Degeneration (CBD) subjects and their use in clinical trials of disease-modifying therapeutics.
MRI & Corticobasal Degeneration (CBD)
Longitudinal MRI brain atrophy measures from the 4RTNI and FTLDNI studies including sample size calculations for clinical trials of Corticobasal Degeneration.
Frontotemporal Dementia (FTD) & MRI Brain Atrophy
Neuroimaging biomarkers (including MRI brain atrophy) from the FTLDNI natural history study of Frontotemporal Dementia (FTD).
Neuroimaging in Frontotemporal Dementia & Clinical Trials
The utility of MRI & PET imaging biomarkers in our understanding of Frontotemporal Dementia (FTD) variants, and their use as endpoints in FTD clinical trials.